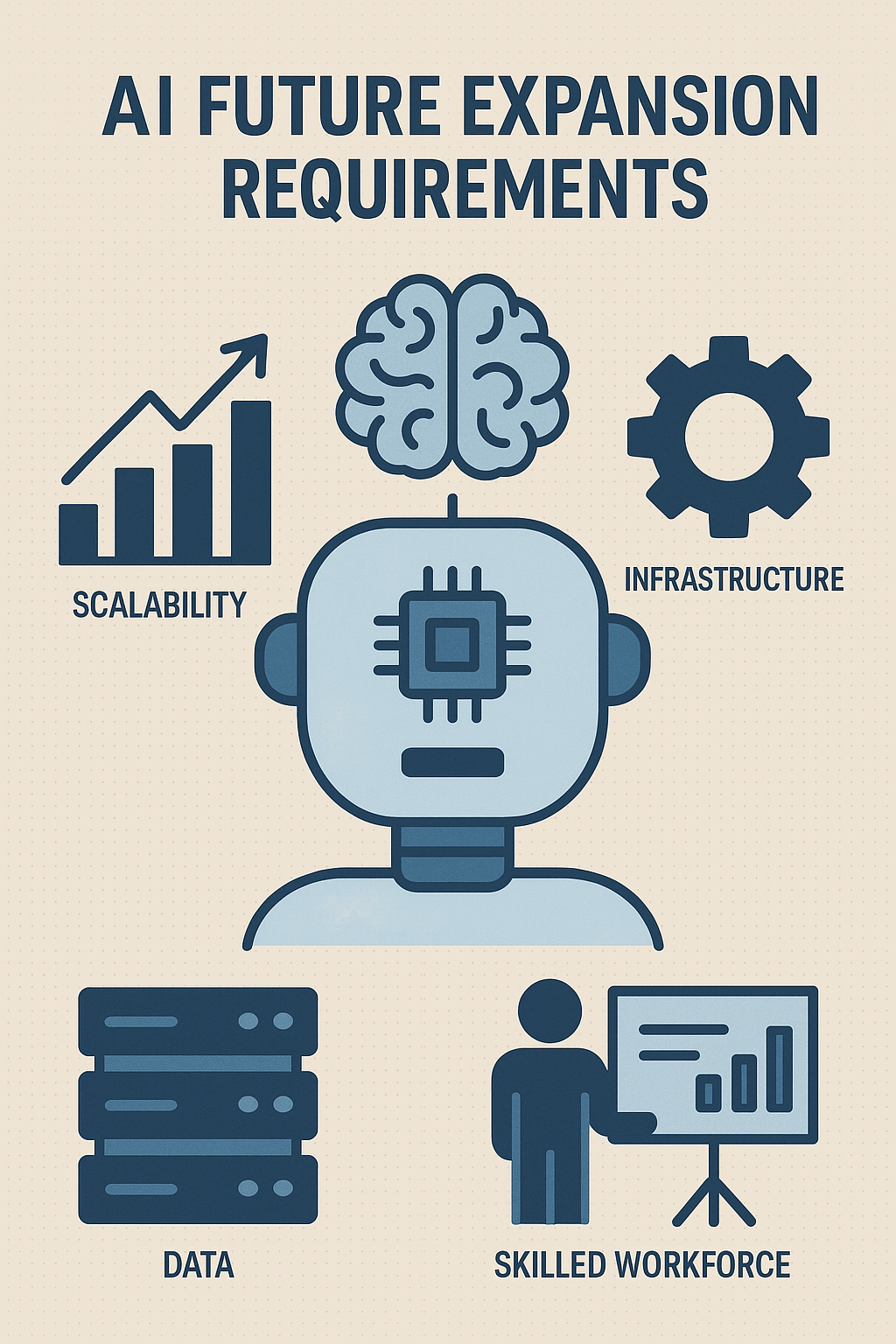
Picture your smartphone struggling to load a simple app. Now imagine trying to run advanced AI systems that could revolutionize healthcare, transform industries, and solve complex global challenges. The gap between these two scenarios illustrates why understanding AI’s future expansion requirements is crucial for anyone invested in technology’s future.
Infrastructure Requirements
The backbone of AI expansion rests on three pillars of infrastructure:
Computing Power and Hardware Needs
- Advanced GPU and TPU architectures capable of handling trillion-parameter models
- Quantum computing integration for specific AI applications
- Specialized AI chips optimized for training and inference tasks
- Distributed computing networks that can scale dynamically
Data Storage and Management Systems
- Petabyte-scale storage solutions with rapid access capabilities
- Real-time data processing pipelines
- Efficient data compression and retrieval algorithms
- Cloud-native architectures supporting global distribution
Network Capabilities and Bandwidth
- 5G and future 6G networks enabling edge AI deployment
- Ultra-low latency connections for real-time AI applications
- Fiber optic infrastructure supporting massive data transfers
- Content delivery networks optimized for AI model distribution
Data Requirements
The fuel for AI’s future growth comes from data, but not just any data:
Quality and Quantity of Training Data
- Datasets must grow from gigabytes to exabytes
- High-quality, labeled data remains a bottleneck
- Synthetic data generation technologies need advancement
- Real-world data collection must scale exponentially
Data Diversity and Representation
- Geographic diversity ensuring global applicability
- Demographic representation preventing bias
- Multi-modal data including text, image, video, and sensor data
- Edge case data capturing rare but important scenarios
Privacy and Ethical Data Collection
- Federated learning architectures preserving privacy
- Differential privacy techniques protecting individual data
- Consent management systems at scale
- Transparent data usage policies and audit trails
Energy and Environmental Considerations
AI’s appetite for power presents significant challenges:
Power Consumption Challenges
- Current large language models consume megawatts during training
- Inference costs multiply with deployment scale
- Cooling requirements add 40-50% to energy needs
- Peak demand management becomes critical
Sustainable Energy Solutions
- Renewable energy integration for data centers
- Energy-efficient hardware design priorities
- Dynamic workload scheduling based on renewable availability
- Power purchase agreements with clean energy providers
Carbon Footprint Reduction Strategies
- Carbon-neutral commitments by 2030-2040
- Efficient model architectures reducing computational needs
- Location optimization for data centers
- Lifecycle assessment of AI hardware
Talent and Workforce Development
Human expertise remains irreplaceable:
AI Researchers and Engineers
- Demand exceeds supply by 10x in specialized roles
- PhD-level expertise requirements for cutting-edge development
- Competitive global talent market
- Remote work enabling broader talent access
Cross-disciplinary Expertise Needs
- Domain experts collaborating with AI specialists
- Ethics and philosophy backgrounds for responsible AI
- User experience designers for AI interfaces
- Policy experts guiding regulation compliance
Training and Education Programs
- University programs adapting to industry needs
- Corporate training initiatives at scale
- Online certification programs democratizing access
- Apprenticeship models bridging academia and industry
Security and Safety Measures
As AI becomes more powerful, security grows critical:
Robust Security Frameworks
- Zero-trust architectures for AI systems
- Encryption of models and data in transit and at rest
- Access control systems with granular permissions
- Threat detection specific to AI attack vectors
AI Alignment and Control Mechanisms
- Value alignment research preventing unintended consequences
- Kill switches and containment protocols
- Human-in-the-loop systems for critical decisions
- Interpretability tools explaining AI decisions
Testing and Validation Protocols
- Rigorous testing environments mimicking real-world conditions
- Adversarial testing identifying vulnerabilities
- Continuous monitoring post-deployment
- Version control and rollback capabilities
Regulatory and Legal Frameworks
Governance structures must evolve rapidly:
Government Oversight and Policies
- National AI strategies guiding development
- Safety standards for critical applications
- Liability frameworks for AI decisions
- Innovation-friendly regulations balancing progress and protection
International Cooperation Standards
- Global AI governance initiatives
- Cross-border data flow agreements
- Harmonized safety standards
- Collaborative research frameworks
Compliance Requirements
- Industry-specific regulations (healthcare, finance, transportation)
- Documentation and audit requirements
- Certification processes for AI systems
- Regular compliance assessments
Business and Economic Considerations
Financial aspects drive practical implementation:
Investment Requirements
- $100+ billion annual global AI investment needed
- Infrastructure costs dominating budgets
- Long-term ROI horizons of 5-10 years
- Public-private partnerships sharing risks
ROI Expectations
- Productivity gains of 20-40% in applicable sectors
- Cost reductions through automation
- New revenue streams from AI-enabled services
- Competitive advantages justifying investments
Market Readiness Factors
- Customer acceptance and trust levels
- Integration with existing systems
- Change management requirements
- Skills availability in target markets
Key Takeaways
Building AI’s future requires addressing multiple interconnected challenges:
- Infrastructure must scale dramatically – Computing power, storage, and networks need exponential growth
- Data requirements go beyond quantity – Quality, diversity, and ethical collection become paramount
- Energy efficiency isn’t optional – Sustainable AI development requires green energy solutions
- Human talent remains crucial – Technical expertise must combine with ethical and domain knowledge
- Security can’t be an afterthought – Robust frameworks must evolve with AI capabilities
- Regulation needs balance – Governance must enable innovation while ensuring safety
- Economics drive adoption – Clear ROI and sustainable business models enable expansion
The path forward demands coordinated efforts across technology companies, governments, academic institutions, and civil society. Success means creating AI systems that are not only powerful but also safe, ethical, and beneficial for humanity. Organizations preparing now for these expansion requirements will lead tomorrow’s AI-powered world.
Unlock your AI Edge — Free Content Creation Checklist
Get the exact AI-powered process to 10X your content output — blogs, emails, videos, and more — in half the time.
No fluff. No spam. Just real results with AI.