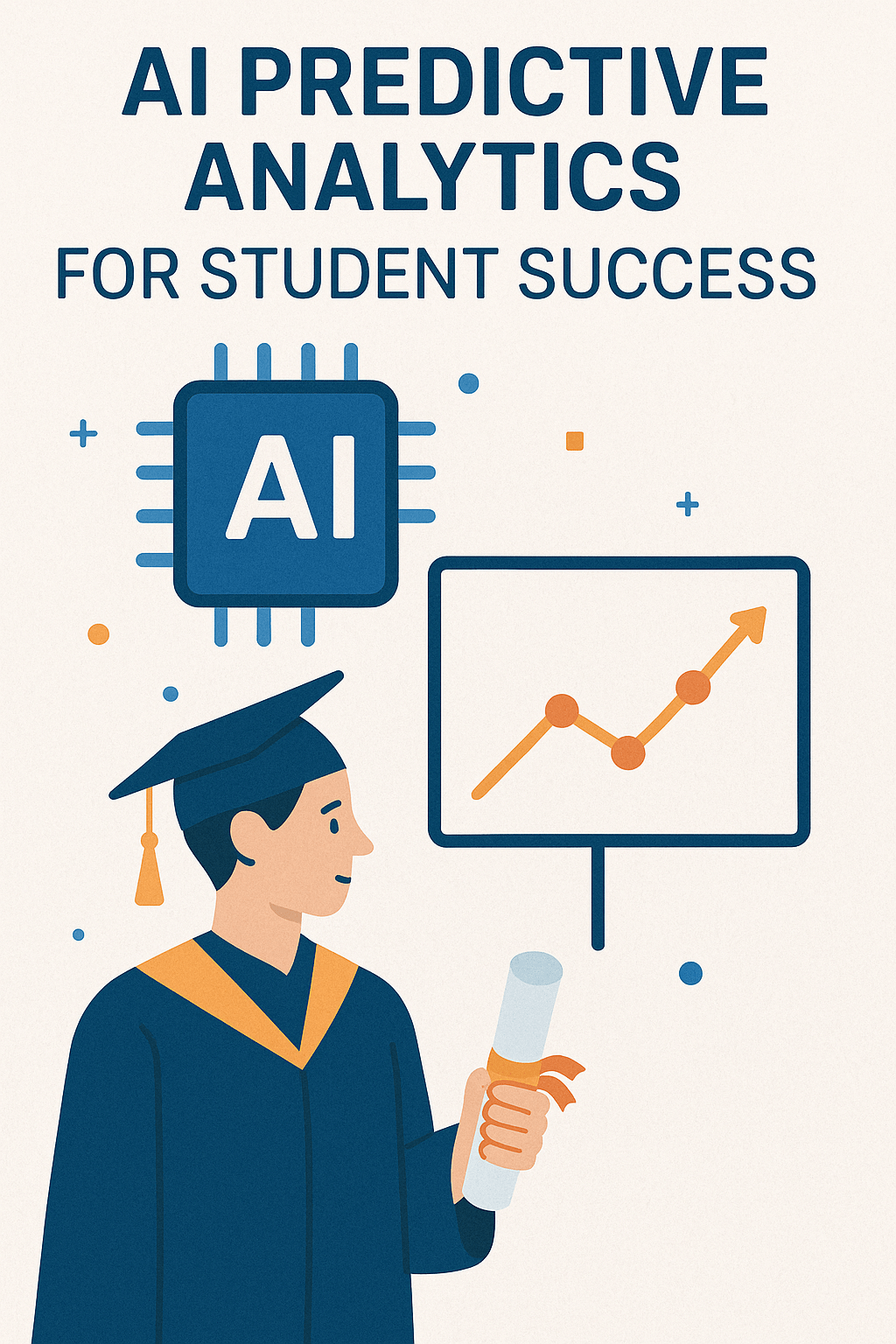
What if you could identify which students would struggle—weeks before they fail an exam? What if you knew exactly which learning resources would help each student most?
This isn’t wishful thinking. It’s happening right now in educational institutions using AI predictive analytics.
The New Reality of Student Success
Traditional education operates largely on intuition and delayed feedback. Instructors often discover student struggles too late—after grades drop or attendance falters. By then, intervention effectiveness plummets.
AI predictive analytics changes this paradigm completely.
What Is AI Predictive Analytics in Education?
AI predictive analytics uses historical and real-time student data to forecast future performance and identify optimal intervention points. It processes vast amounts of information no human could reasonably analyze, spotting patterns invisible to even the most attentive educators.
Key Components:
- Data Collection – Academic records, attendance, LMS engagement, demographic information
- Pattern Recognition – Machine learning algorithms identifying correlations between behaviors and outcomes
- Actionable Insights – Specific recommendations for interventions tailored to individual students
- Continuous Improvement – Systems that learn and improve with each new cohort
How These Systems Actually Work
Unlike basic analytics that simply report what happened, predictive systems apply sophisticated machine learning models to forecast what will happen.
The Technical Foundation:
- Supervised Learning Models – Trained on historical student data to identify success and failure patterns
- Natural Language Processing – Analyzing student writing for comprehension indicators
- Time Series Analysis – Tracking patterns of engagement across semesters
- Classification Algorithms – Categorizing students by risk levels and intervention needs
What These Systems Track:
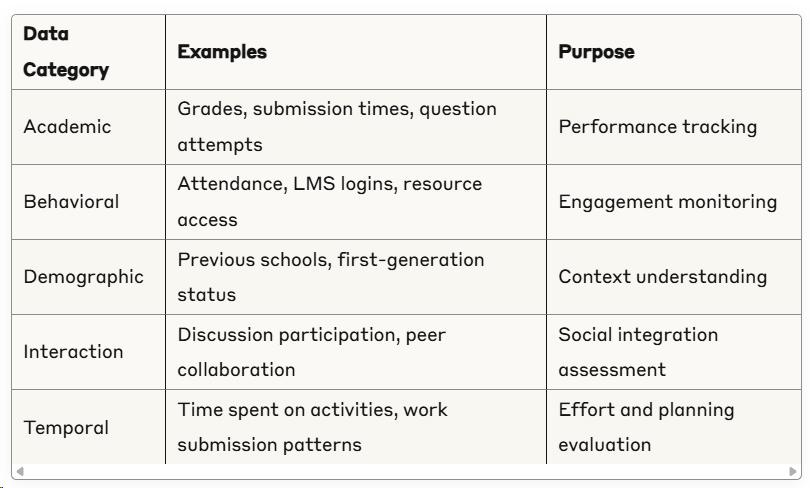
Real Applications Transforming Education Now
1. Early Warning Systems
AI systems can flag at-risk students with 85-95% accuracy, often 3-4 weeks before traditional methods would identify problems.
How it works: Systems analyze LMS logins, assignment completion patterns, and early assessment scores to identify struggling students before midterm exams.
Results: One community college system reduced dropout rates by 16% after implementing AI early warning tools.
2. Personalized Learning Pathways
AI doesn’t just identify problems—it can prescribe solutions tailored to each student’s needs.
How it works: After identifying knowledge gaps, systems recommend specific resources, practice activities, or alternative learning approaches based on individual learning patterns.
Results: A study of 8,000 students using AI-recommended learning paths showed a 23% improvement in concept mastery compared to control groups.
3. Resource Allocation Optimization
For administrators, predictive analytics transforms how resources are deployed.
How it works: AI forecasts which courses will have high failure rates, where tutoring will yield the greatest improvement, and which faculty interventions produce the best outcomes.
Results: One university reduced academic probation rates by 35% while simultaneously reducing tutoring costs by reallocating resources to highest-impact areas.
4. Curriculum Development and Refinement
Identify exactly where learning materials fall short.
How it works: AI systems identify specific concepts, questions, or materials that consistently correlate with student confusion or misunderstanding.
Results: A biology department improved pass rates by 18% after using AI to identify and redesign five key concept explanations that were causing student confusion.
Implementation: Making It Work in Your Institution
Essential Infrastructure Requirements
- Data Integration Platform – Connecting siloed systems (SIS, LMS, etc.)
- Secure Data Warehouse – FERPA-compliant storage and processing
- Visualization Tools – Dashboards for administrators, faculty, and students
- Intervention Management System – Tracking which interventions work for whom
Integration Strategy
Successful implementation follows this pathway:
- Audit existing data sources – Identify what you’re already collecting
- Start with a focused use case – Early warning system for high-risk courses
- Build faculty buy-in – Demonstrate value through pilot programs
- Scale gradually – Expand based on proven successes
- Create feedback loops – Continuously improve models with new data
Overcoming Common Challenges
Data Quality and Access
Challenge: Fragmented, inconsistent, or missing student data.
Solution: Begin with data cleaning initiatives and implement standard data collection protocols. Start prediction models with the most reliable data points, then expand.
Privacy and Ethics
Challenge: Balancing analytical power with student privacy and avoiding bias.
Solution:
- Create clear data governance policies
- Implement differential privacy techniques
- Establish ethics committees to review algorithms
- Focus on actionable insights rather than predictions alone
Faculty Adoption
Challenge: Resistance to technology-driven decision-making.
Solution: Position AI as a tool to amplify faculty expertise, not replace it. Provide robust training and start with faculty champions who can demonstrate success.
Real-World Success Stories
Arizona State University
ASU implemented predictive analytics to identify optimal course paths. Their system analyzes over 800 million potential combinations of courses, timing, and sequencing.
Results:
- 400% increase in four-year graduation rates
- Saved students an average of $21,000 in tuition costs
- Reduced time-to-degree by 2.3 semesters on average
Georgia State University
GSU uses a predictive analytics system that identifies 800+ risk factors for student dropout.
Results:
- Eliminated achievement gaps based on race, ethnicity, and socioeconomic status
- Improved six-year graduation rates by 23 percentage points
- Generated 2,000+ daily alerts for advisors to intervene with specific students
The Future of AI in Education
The next wave of predictive analytics will feature:
- Multimodal Analysis – Incorporating video, audio, and physical classroom behavior
- Emotion Recognition – Identifying student frustration, engagement, or confusion in real-time
- Lifelong Learning Models – Systems that follow students from K-12 through higher education and career
- Collaborative Intelligence – AI and human educators working symbiotically rather than separately
Getting Started: Your Action Plan
Step 1: Assessment (Weeks 1-4)
- Inventory existing data systems and quality
- Identify high-priority student success challenges
- Form cross-functional implementation team
Step 2: Planning (Weeks 5-8)
- Select initial use case (recommendation: early warning system)
- Define success metrics
- Determine technology requirements
Step 3: Pilot Implementation (Months 3-6)
- Deploy in limited courses or departments
- Train faculty and staff
- Establish baseline measurements
Step 4: Evaluation and Expansion (Months 7-12)
- Assess impact against success metrics
- Refine models based on feedback
- Develop plan for expanded implementation
Key Resources to Get Started
- Data Assessment Tools
- Educational Data Mining Society resources
- Institution-specific data auditing frameworks
- Vendor Evaluation Framework
- Requirements checklist for predictive analytics platforms
- Integration capability assessment
- Faculty Training Materials
- Data literacy fundamentals
- Intervention best practices
Final Thoughts: Beyond the Algorithm
The most successful implementations of AI predictive analytics share one critical feature: they focus on human action, not just prediction accuracy.
The best system in the world provides zero value if insights don’t translate to interventions. Build your strategy around the human elements—faculty training, student communication, and intervention resources.
When AI prediction meets human connection, that’s when student success truly transforms.
Are you ready to move beyond hindsight and gut feelings to a data-informed approach to student success? The technology exists today—and your students deserve nothing less.
Unlock your AI Edge — Free Content Creation Checklist
Get the exact AI-powered process to 10X your content output — blogs, emails, videos, and more — in half the time.
No fluff. No spam. Just real results with Ai.